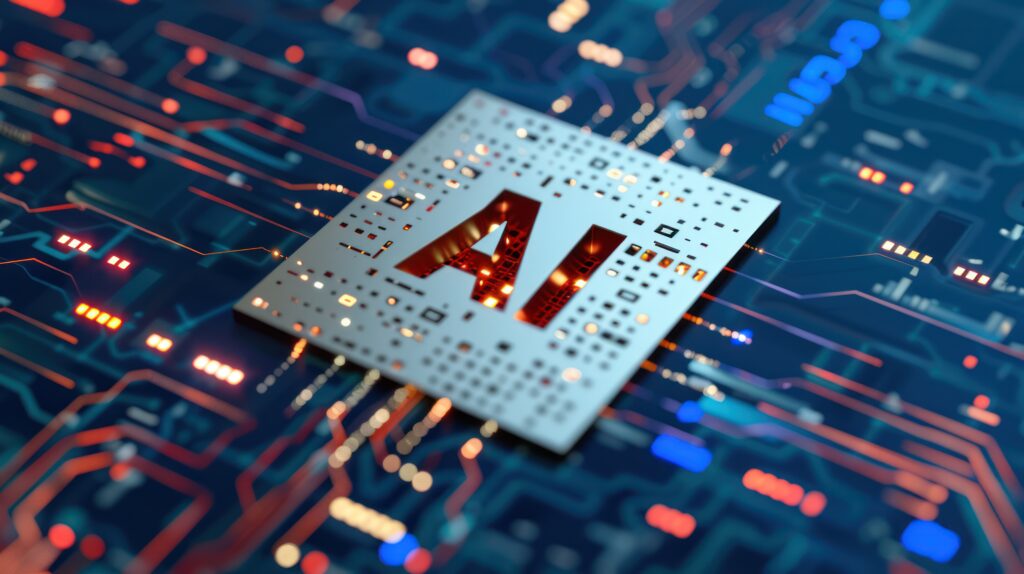
Artificial Intelligence (AI) has gone from being a tech buzzword to an indispensable tool in IT infrastructure management.
By automating repetitive tasks, optimizing systems, and predicting failures before they occur, AI is reshaping how IT departments operate. Gone are the days when IT teams waited for outages to pounce like unwelcome surprises—AI’s proactive approach ensures smoother, more efficient management.
How AI is Transforming IT Operations
Automation of Routine Tasks
AI-driven IT automation is a game changer for IT operations, handling repetitive and time-consuming tasks with ease. Routine maintenance tasks, such as updating software patches, monitoring server health, and even resetting user passwords, can now be fully automated. This doesn’t just free up valuable human resources—it also ensures tasks are executed consistently without the risk of human error.
Take AI-driven monitoring systems as an example. These tools don’t just detect performance anomalies; they also prioritize them based on severity. For instance, if one system flag shows a temporary CPU spike while another reveals a critical memory leak, AI can determine which issue poses a greater risk and alert the IT team accordingly. This proactive prioritization means teams can focus their energy where it’s most needed, rather than wading through endless logs.
Another key feature is automation in ticketing. When an issue arises, AI systems can create and categorize tickets based on the type and urgency of the problem. This removes the need for manual triage, which is not only labor-intensive but often leads to delays. Imagine an AI system recognizing a failing disk in a server, generating a ticket, assigning it to the appropriate technician, and even including potential solutions—all without human intervention.
Example in Action: The Commonwealth Bank of Australia implemented an AI-driven alert and investigation management system to streamline its processes for detecting and managing financial crime alerts. By integrating functions previously handled by multiple applications, the AI system reduced manual labor and enhanced decision-making. It allowed the bank to process alerts more quickly, identify patterns through visual transaction maps, and improve operational efficiency, showcasing the transformative power of AI in complex environments.
Enhanced Decision-Making
Modern IT infrastructure generates an overwhelming amount of data daily, from server logs to application performance metrics. For humans, sifting through this data manually is akin to finding a needle in a haystack. AI-powered tools not only process this data at lightning speed but also extract actionable insights, making decision-making more efficient and precise.
AIOps platforms are leading this transformation by going beyond data collection. These systems analyze historical data to establish baselines and recognize deviations that could signal potential vulnerabilities. For instance, if a server typically runs at 60% CPU utilization but suddenly spikes to 90%, the AI doesn’t just flag the anomaly—it correlates it with related events, such as a new application deployment or increased user load. This context allows IT leaders to diagnose issues faster and with greater accuracy.
AI tools also excel at scenario planning. By simulating the impact of different decisions—like increasing server capacity or migrating workloads to the cloud—AI enables IT teams to weigh potential outcomes before taking action. This reduces the risk of costly missteps.
Example in Action: Imagine a mid-sized enterprise facing challenges with resource allocation during fluctuating demand periods. By utilizing an AI-powered capacity planning tool, they analyze historical usage patterns to forecast future resource needs accurately. This proactive approach helps them avoid over-provisioning during peak traffic periods while ensuring sufficient resources are available when demand spikes. With such optimization, they could potentially save up to 25% on cloud costs over a year.
Real-World Insight: IBM has developed an AI-driven system that enhances IT security by prioritizing patches based on the likelihood of exploitation and potential impact. This system analyzes extensive data, including historical attack patterns and current threat intelligence, to identify vulnerabilities. By focusing on the most critical security issues, IBM’s AI solutions help IT leaders allocate resources efficiently, reducing the risk of breaches while improving overall security management.
The Benefits of AI in Infrastructure Management
Reduction in Downtime
AI for reducing IT downtime is one of its most compelling features, with its ability to predict and prevent system failures.. By analyzing historical performance data and spotting irregularities, AI can alert teams to potential issues before they cause outages. Think of it as the meteorologist for your IT environment, predicting storms so you can take out your umbrella well in advance.
Beyond just alerting teams, AI can often take preventive actions itself, such as reallocating resources, restarting services, or rerouting traffic to mitigate impending failures. For instance, in network environments, AI can detect early signs of latency and automatically reroute data to maintain performance. This proactive management not only prevents downtime but also enhances user experience, ensuring that end-users are largely unaware of any behind-the-scenes issues.
Cost Efficiency
AI in IT cost optimization plays a key role by automating manual tasks and optimizing resource allocation, reducing the need for constant human intervention while saving time and labor costs. Moreover, it minimizes over-provisioning by ensuring systems scale based on real demand—your budget will thank you.
AI can also identify inefficiencies within existing operations, such as underutilized servers or redundant processes, and recommend cost-saving changes. In cloud environments, AI-driven tools can optimize storage and compute costs by shifting workloads to lower-cost resources or automatically shutting down unused instances. These savings can be reinvested into other areas, such as innovation or scaling, helping companies make the most of their IT budgets.
Improved Scalability
With AI tools, IT systems can dynamically adjust to fluctuating workloads, scaling up or down as needed. This is crucial for companies managing high-demand applications or seasonal traffic surges. AI ensures you’re not stuck paying for unused capacity or scrambling for extra resources during a spike.
Scalability powered by AI also enables businesses to enter new markets or handle unpredictable growth more confidently. For example, e-commerce companies experiencing sudden surges during flash sales or holiday shopping events can rely on AI to ensure their systems scale appropriately, avoiding potential website crashes. This flexibility allows organizations to maintain optimal performance levels without overburdening IT teams or overspending on fixed resources.
Predictive Maintenance and Failure Prevention
AI’s predictive capabilities are redefining IT infrastructure management. Using machine learning models, AI analyzes performance data, system logs, and user activity to identify patterns that precede failures. Whether it’s a server showing early signs of wear or a configuration issue in your network, AI spots the problem before it causes disruption. This proactive approach means fewer panicked late-night calls and more strategic IT planning.
What makes predictive maintenance especially powerful is its ability to prioritize actions based on potential impact. For instance, if multiple alerts are flagged, AI can rank them by severity and recommend which issues require immediate attention. This ensures IT teams focus on critical tasks first, rather than getting bogged down in minor concerns. Additionally, the insights gathered from predictive tools can inform long-term maintenance schedules, reducing the likelihood of recurring issues and extending the lifespan of infrastructure components.
Challenges of AI Adoption in IT
Despite its advantages, implementing AI isn’t without hurdles:
Initial Investment Costs
Deploying AI tools can be pricey, especially for smaller organizations. Convincing leadership to greenlight these investments often requires showing tangible ROI—like fewer outages and lower operational costs. It’s a bit like trying to sell the idea of a self-cleaning coffee machine: sure, it’s a big upfront cost, but no one’s going to miss scrubbing out the coffee pot every morning. However, the cost challenge extends beyond just the initial purchase of AI tools. Companies must also factor in expenses related to integrating AI with existing systems, hiring or upskilling employees, and ongoing maintenance. These hidden costs can make AI adoption seem daunting to organizations already operating within tight budgets.
One way to address this is by starting with a phased approach—implementing AI for specific high-impact use cases first, such as automating repetitive tasks or optimizing resource allocation. By demonstrating measurable benefits in these smaller projects, IT leaders can build a case for broader AI adoption. It’s like convincing the CFO one coffee pot at a time; once they see the magic of automated results, the financial investment becomes a much easier pill to swallow.
Learning Curve
IT teams need time and training to adapt to new AI-powered tools. It’s not as simple as flipping a switch; understanding how to integrate these systems into existing workflows is crucial. Beyond technical skills, teams must also develop new ways of thinking, shifting from reactive problem-solving to proactive management using AI insights. This cultural shift can be as challenging as the technical learning curve itself.
For instance, while AI can identify anomalies or suggest optimizations, IT teams must still interpret these findings and act on them appropriately. Without proper training, there’s a risk of either over-relying on AI or underutilizing its capabilities, reducing its effectiveness. Organizations can mitigate this by offering structured training programs, creating AI “champions” within teams to lead the charge, and fostering an environment of continuous learning.
Data Quality
AI’s effectiveness depends on the quality of the data it’s fed. Messy, incomplete, or outdated data can lead to inaccurate predictions and insights, undermining its potential. For instance, if an AI system is tasked with predicting system failures but is working with inconsistent performance logs, it may either miss critical issues or trigger false alarms, frustrating IT teams and reducing trust in the tool.
Addressing this challenge requires a robust data governance strategy. Organizations must prioritize collecting clean, consistent data and invest in tools or processes to standardize it. This may include consolidating disparate data sources, implementing data validation practices, and ensuring regular audits to maintain data quality. While it’s a time-consuming process, the payoff is significant: accurate insights, actionable recommendations, and AI systems that deliver on their promises.
The Future of AI in IT Infrastructure Management
As AI advances, the dream of fully autonomous IT infrastructure management is becoming a reality. Imagine a system that not only detects and resolves issues but also optimizes itself continuously. IT teams may soon shift from firefighting problems to designing and supervising high-level strategies. Instead of spending hours troubleshooting network glitches, they’ll be strategizing how to streamline operations further—or maybe just enjoying lunch without interruption for once.
These systems could take automation to the next level by dynamically adjusting resource allocation, updating configurations, and even self-healing after minor errors—all without human intervention. Think of it as the autopilot of IT: reliable, efficient, and always on. Sure, humans will still need to oversee the process, but their roles will shift toward innovation and oversight rather than monotonous tasks. It’s the IT dream—less time with tedious logs, more time designing the next big thing.
AI-Powered Security
AI isn’t just a tool for managing infrastructure—it’s becoming a critical component in cybersecurity. By detecting unusual activity patterns and predicting potential breaches, AI adds another layer of defense to IT systems. Picture an AI-powered security system like a guard dog for your network, constantly sniffing out intruders while you sleep. Except, this guard dog doesn’t bark—it sends you detailed reports with actionable insights.
Beyond detecting threats, AI can also automate responses, such as blocking suspicious IPs or isolating infected systems to prevent further spread. For example, AI can identify ransomware activity within seconds, quarantining the threat before it encrypts your entire database. It’s not just about protection—it’s about speed and precision, qualities that make AI an invaluable partner in cybersecurity. And best of all? It doesn’t ask for coffee breaks.
Key Takeaways
- Proactive, Not Reactive: AI transforms IT management from a reactive to a proactive process, predicting and preventing failures before they occur—saving teams from panicked, late-night troubleshooting sessions.
- Efficiency and Savings: Automation reduces downtime, operational costs, and over-provisioning, allowing IT teams to focus on strategy rather than firefighting.
- Challenges Exist: Initial costs, steep learning curves, and the need for clean, reliable data must be addressed for successful AI adoption. Even the smartest AI can’t fix garbage input.
- Bright Future: Autonomous systems and AI-powered security promise a future where IT teams supervise innovation, not troubleshoot chaos, while networks practically guard themselves.
AI may not replace IT teams, but it’s certainly redefining their roles. By automating the mundane and enhancing strategic decision-making, AI ensures that the IT department isn’t just putting out fires—it’s building a foundation for growth and innovation.